Write-through caching is a caching mechanism where data modifications are written to both the source database and the cache. In this approach, every write operation triggers a write to a RAM cache (such as redis) and the source database (such as postgres).
This is commonly used to complement look-aside caching pattern so that when subsequent read operations request the same data, there’s a greater likelihood of a cache-hit, therefore reducing the response time and minimizing the load on the source data store. Additionally, the cached data being returned will be more fresh compared to a strategy that only uses TTL or expiration based invalidation.
The key benefit to using a write-through cache is data freshness. By updating the cache during updates to the source database, you ensure that the cached data remains up to date. One of the common problems with relying strictly on expiry based invalidation to serve fresh data is the risk of stale data during the period between when the data is cached and when it expires.
How to implement (Ruby on Rails Example)
Lets look at a simple example. When data needs to be written, it is first updated in the source database and then persisted in the cache. Here’s an example using rails. In this example, we’re reading a list of blog posts using look-aside caching. On writes (updates to an individual blog post), we update the cache. This keeps the list of blog posts in the cache fresh!
class PostsController < ApplicationController
def index
@posts = Rails.cache.fetch('posts', expires_in: 1.hour) do
Post.all.to_a
end
render json: @posts
end
def create
@post = Post.new(post_params)
if @post.save
update_posts_cache
render json: @post, status: :created
else
render json: @post.errors, status: :unprocessable_entity
end
end
def update
@post = Post.find(params[:id])
if @post.update(post_params)
update_posts_cache
render json: @post
else
render json: @post.errors, status: :unprocessable_entity
end
end
def destroy
@post = Post.find(params[:id])
@post.destroy
update_posts_cache
head :no_content
end
private
def post_params
params.require(:post).permit(:title, :content)
end
def update_posts_cache
Rails.cache.write('posts', Post.all.to_a, expires_in: 1.hour)
end
end
In this example, the index
action retrieves the list of blog posts from the cache using the key `posts
`. If the cache contains the posts, they are returned directly. Otherwise, the posts are fetched from the database (Post.all.to_a
) and stored in the cache with a 1-hour expiration time.
Implementing write-through isn’t always straightforward because cached data may not always be easily re-computed on writes. The extent to which you can re-compute cached data during a write greatly affects the feasibility of a write-through implementation. In the example above, we re-computed the list of posts in the cache by invoking Posts.all.to_a
in the code for writing. But what if instead of serving all posts on reads, our application only serves unread posts for logged in users? If you have 10 logged in users, they need to be served different lists of posts! (As an exercise, think about how you would handle this!).
Sometimes cache re-computability is affected by the API paradigm you choose. RESTful services are able to leverage write through caching better than GraphQL services. Lets see why.
GraphQL and Write Through Caching
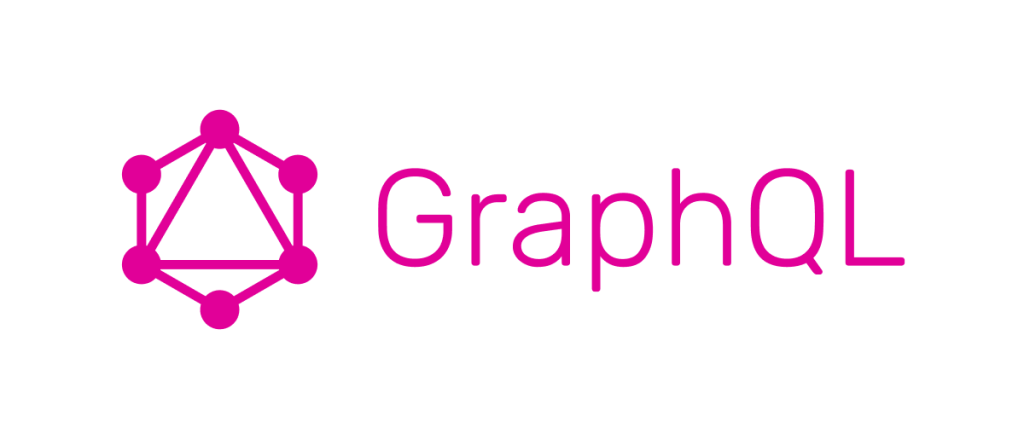
At work we heavily use GraphQL API’s and GraphQL presents unique challenges for caching. The entire paradigm of GraphQL rests on allowing clients to query for the data they need. This leads to a higher variability in queries of nearly unbounded complexity (you can have highly nested queries). Common caching techniques can be easily applied to applications serving a small set of frequently executed but fixed queries – but if every query is likely going to be unique, the value of caching is diminished.
There’s a bigger reason though why caching (particularly write-through caching) is trickier with GraphQL. In reality, most GraphQL servers are built for internal product teams and client queries aren’t infinitely variable. There’s typically some fixed number of clients that use some set of queries to power an experience.
For example, at a video streaming company there may be a recommendations team (the client) that uses a set of queries for fetching video metadata from an internal video catalog service (graphql server).
Lets assume…
- There’s a high traffic query that looks like this defined within the recommendation client:
query { videos { title createdAt reviews { reviewerFullName } } }
. - Within the video service, query results are cached on read with look-aside caching.
Now lets say you want to implement write-through caching to keep the cache of the recommendation teams query results warm. If video metadata gets updated throughout the day in the video catalog service (lets just say by some other service via HTTP), how does the cache get updated?
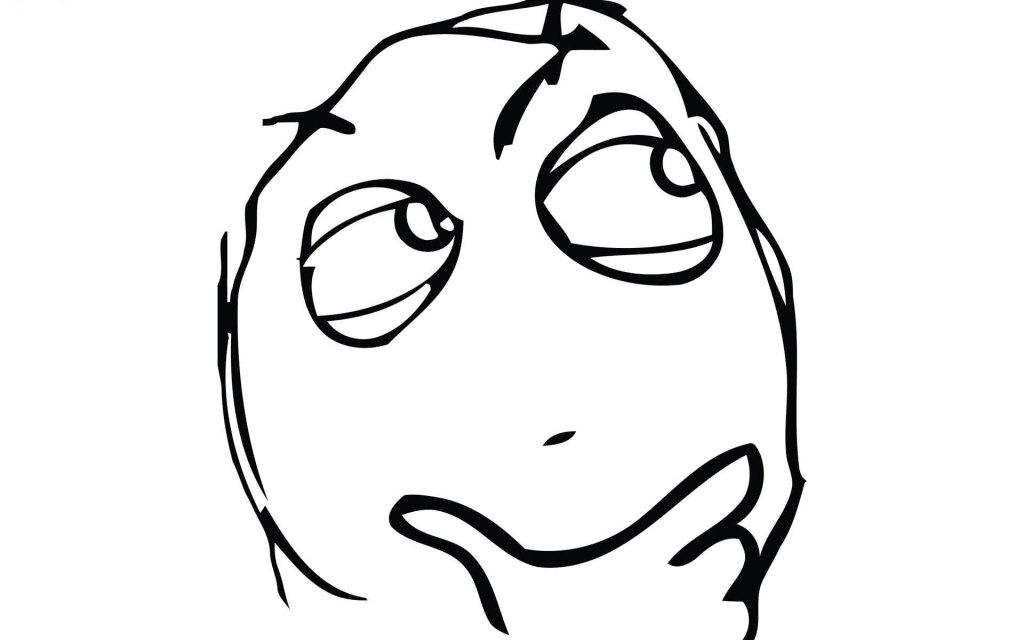
Here’s what we know:
- The query is defined on the client (unlike REST, where the query is defined on the server).
- The result of the query operation is computed at runtime in the video service resolvers.
This is a situation where the cached data (JSON result of the GraphQL query) is no longer easily computable. In order to compute that data, you need to execute the original query against the schema using the same inputs. But wait, GraphQL servers don’t define the queries, clients do! 😞
Unless the application changes the type of data that gets cached or the server records queries being executed against the API (to be “replayed” on relevant writes), warming this type of cache using write-through is not really possible. So in practice, most GraphQL servers focus on lazy-loading read-based caching strategies such as automatic persisted queries using GET requests or fragment caching on the server side.
Risks
Adopting write-through caching isn’t without risks – before implementing it, it’s worth considering the following in the context of your unique problem.
- Increased Write Latency. Write-through caching introduces additional overhead due to the need to write data to both the cache and the main data store before returning a result. This can be an issue for applications with high writes that cannot tolerate additional write latency. That said, most users of applications generally have higher tolerance for writes than for reads.
- Redundant / Unnecessary data in cache. The cache may fill up regardless of whether the data being written is going to be used. Data being written isn’t really a guarantee of a future read, so you may end up with a cache filled with unread data (which defeats the purpose of a cache). If you don’t have proper eviction policies in place, systems with high write volumes with large amounts of data being written can rapidly fill up a cache and make it unusable. On the other hand, if there are eviction policies in place and you haven’t properly sized your cache, you end up with churn in your cache where data being written to the cache is evicted before it has a chance to be used by readers.
- Delayed benefit. The cache is only updated when something changes as a result of a write. If nothing changes, the cache stays empty. This is also why you rarely see systems that rely exclusively on write-through caching. If updates are infrequent, the cache will stay cold forever unless you also cache when the data is being read.